By Austin Weber // Senior Editor // webera@bnpmedia.com
New System Diagnoses EV Battery Health
AV/EV tech trends
Electrochemical impedance spectroscopy technology can be used to improve the stability and performance of high-capacity batteries in electric vehicles. Illustration courtesy Korea Advanced Institute of Science & Technology
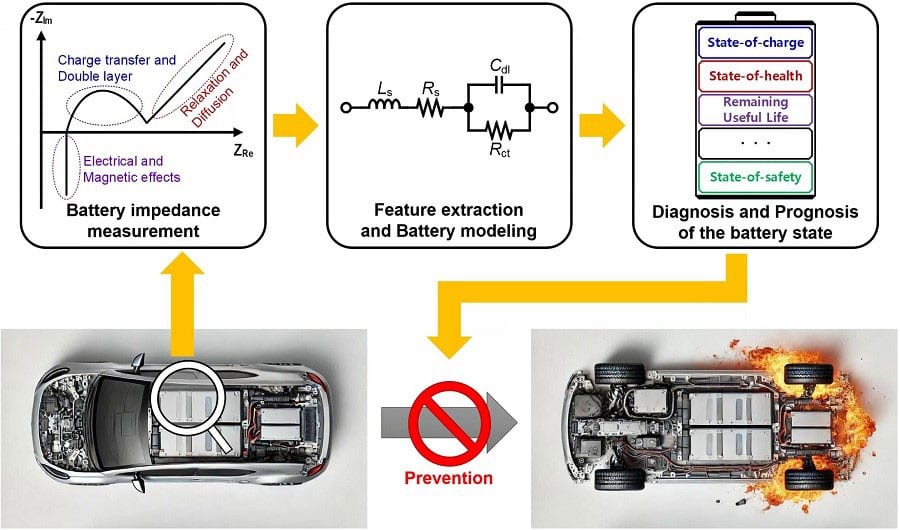
Engineers at the Korea Advanced Institute of Science & Technology (KAIST) have developed a way to precisely determine the health of batteries by only using small amounts of electrical current. Their electrochemical impedance spectroscopy (EIS) technology may improve the stability and performance of high-capacity batteries in electric vehicles.
In addition to assessing the state of charge and state of health of batteries, the tool can be used to identify thermal characteristics, chemical or physical changes, predict battery life and determine the causes of failures.
Traditional EIS equipment is expensive and complex, making it difficult to install, operate and maintain. And, due to sensitivity and precision limitations, applying current disturbances of several amperes to a battery can cause significant electrical stress, increasing the risk of battery failure or fire.
The KAIST system can precisely measure battery impedance with low current disturbances, minimizing thermal effects and safety issues during the measurement process. It minimizes bulky and costly components, making it easy to integrate into EVs.
The system was proven effective in identifying the electrochemical properties of batteries under various operating conditions, including different temperatures and state of charge levels.
“This system can be easily integrated into the battery management system of electric vehicles and has demonstrated high measurement accuracy, while significantly reducing the cost and complexity compared to traditional high-current EIS methods,” says Kyeongha Kwon, Ph.D., an electrical engineering professor at KAIST. “It can contribute to battery diagnosis and performance improvements not only for electric vehicles, but also for energy storage systems.”
AI Technology Protects Connected Vehicles From Cyberattacks
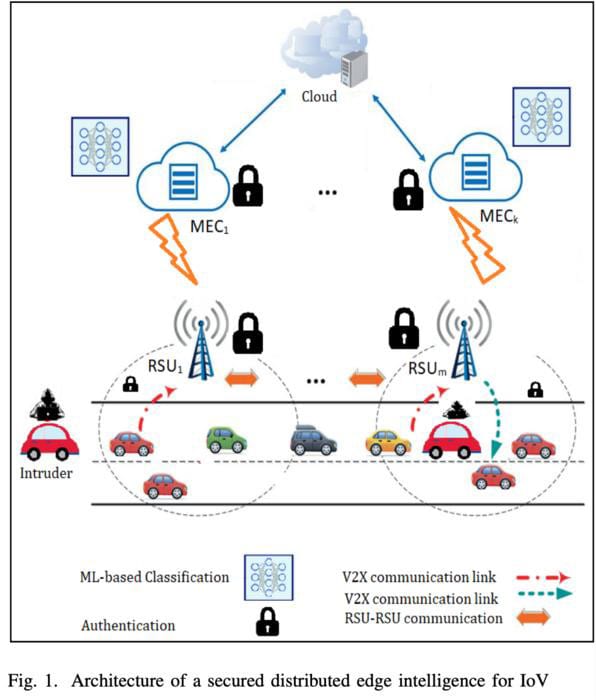
Engineers are using artificial intelligence technology to develop reliable authentication schemes designed to combat possible cyberattacks. Illustration courtesy University of Sharjah/IEEE
The Internet of Vehicles (IoV) refers to a real-time network in which vehicles can communicate with each other, as well as with intelligent communication devices in parking lots, pedestrians and road infrastructure. Vehicles connected via IoV are also equipped with embedded sensors and units that collect useful data and communicate it to the closest roadside units or server modules.
However, limited resources of many onboard units and the shortcomings of embedded sensors installed in connected vehicles pose potential cyberattack risks. To address the issue, engineers at the University of Sharjah are using artificial intelligence technology to develop reliable authentication schemes designed to combat possible attacks.
“The operational capabilities of these vehicles are further augmented by artificial intelligence, particularly machine learning and deep learning, which analyze and interpret data in real-time,” says Mian Ahmad Jan, Ph.D., an assistant professor of computer science at the University of Sharjah, who is working on the project.
According to Jan, the security of vehicles equipped with IoV technology are vulnerable to cyberattacks. That’s because communication systems can encounter challenges related to bandwidth scarcity and delays in the responses from cloud-located services within a stipulated time.
Jan and his colleagues claim that current embedded sensors and processing units are still “unable to provide swift responses” and “unable to support computationally complex security and privacy preservation schemes.”
To address these challenges, the engineers are developing a machine learning-based authentication scheme that “trains and classifies the vehicles at the edge servers in a distributed manner, preserves the privacy of communicating entities, and minimizes bandwidth consumption and delay.”
“Simulation results have concluded that the proposed scheme is not only pruned against well-known intruder attacks, but it is equally lightweight and effective concerning various performance evaluation metrics such as computation, communication and storage overheads,” say Jan.
“The ML-based approach extends the decision power of vehicles and edge servers to identify adversaries,” explains Jan. “Our [system] requires that each vehicle participates in an offline phase, where a trusted authority shares a list of MaskIDs and secret keys of legitimate vehicles and edge servers.”
When a vehicle starts to communicate, the nearest edge server verifies its identity, reducing the computational load on its system.
Machine Learning Speeds Development of Sodium-Ion Batteries
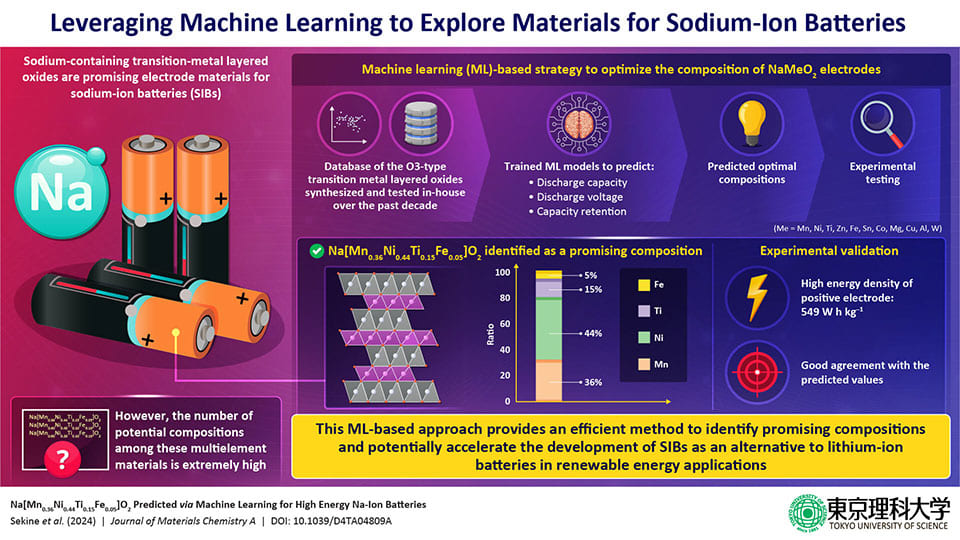
The composition of a multi-element transition metal oxide can produce exceptional energy density in sodium-ion batteries. Illustration courtesy Tokyo University of Science
Engineers around the world are scrambling to develop new types of electric vehicle batteries that don’t depend on lithium and other scarce materials. Sodium-ion batteries are one promising alternative, due to the abundance of sodium, its higher safety and lower cost.
In particular, sodium containing transition-metal layered oxides are powerful materials that could be used to make electrodes, because they offer exceptional energy density and capacity.
However, for multi-element layered oxides composed of several transition metals, the sheer number of possible combinations makes finding the optimal composition both complex and time-consuming. Even minor changes in the selection and proportion of transition metals can bring about marked changes in crystal morphology and affect battery performance.
Engineers at the Tokyo University of Science (TUS) are using machine learning technology to streamline the search process. They recently assembled a database of 100 samples containing 68 different compositions.
"The database includes the composition of sodium transition-metal layered oxides…as well as the upper and lower voltage limits of charge-discharge tests, initial discharge capacity, average discharge voltage and capacity retention after 20 cycles," says Shinichi Komaba, Ph.D., a chemistry professor at TUS.
Komaba and his colleagues are using the database to train a model incorporating several machine learning algorithms, as well as Bayesian optimization, to perform an efficient search. Their goal is to learn how properties like operating voltage, capacity retention and energy density are related to the composition of sodium containing transition-metal layered oxides, and to predict the optimal ratio of elements needed to achieve a desired balance between these properties.
After analyzing the initial results, they assembled standard coin cells to run charge-discharge tests. The measured values were, for the most part, consistent with the predicted ones, highlighting the accuracy of the model and its potential for exploring new battery materials.
"The number of experiments can be reduced by using machine learning, which brings us one step closer to speeding up and lowering the cost of materials development,” explains Komaba. “Furthermore, as the performance of electrode materials for sodium-ion batteries continues to improve, it is expected that high-capacity and long-life batteries will become available at lower cost in the future.”
DECEMBER 2024 | ASSEMBLYMAG.com
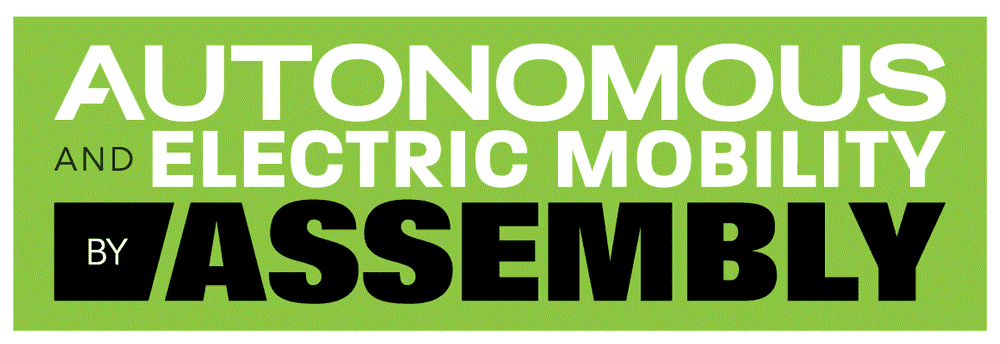