ASSEMBLY LINES
A Faster, Better Way to Train Robots
CAMBRIDGE, MA—Inspired by large language models, engineers at the Massachusetts Institute of Technology (MIT) have developed a unique way to teach general-purpose robots new skills. They created a versatile technique that combines a huge amount of heterogeneous data from many sources into one system that can teach any robot a wide range of tasks.
Their method involves aligning data from varied domains, like simulations and real robots, and multiple modalities, including vision sensors and robotic arm position encoders, into a shared “language” that a generative AI model can process.
By combining such an enormous amount of data, this approach can be used to train a robot to perform a variety of tasks without the need to start training it from scratch each time.
This method could be faster and less expensive than traditional techniques because it requires far fewer task-specific data. In addition, it outperforms training from scratch by more than 20 percent in simulation and real-world experiments.
A new system developed at MIT uses artificial intelligence technology to teach general-purpose robots new skills. Photo courtesy Massachusetts Institute of Technology
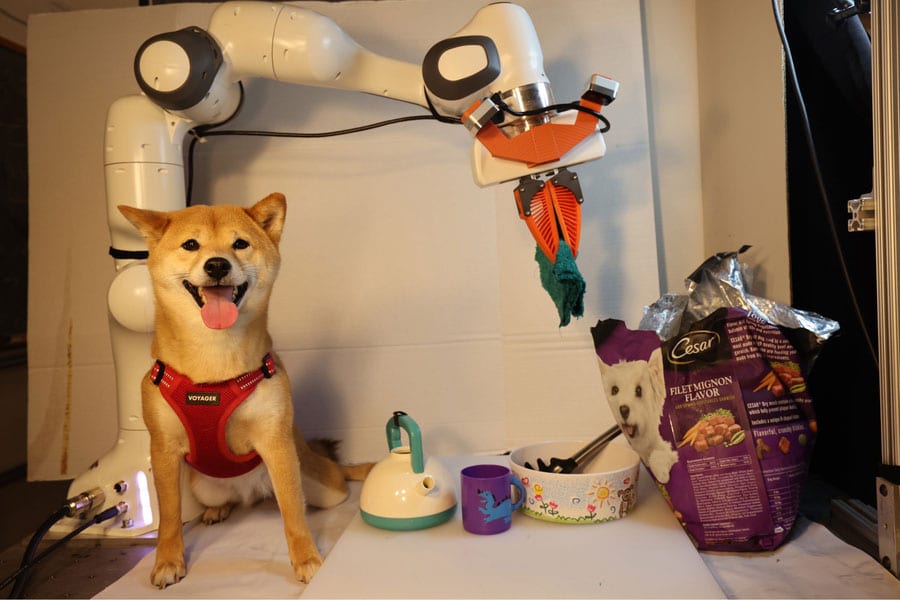
Typically, engineers collect data that are specific to certain robots and tasks, which they use to train the machine in a controlled environment. However, gathering this information is costly and time-consuming, and a robot will likely struggle to adapt to environments or tasks it hasn’t seen before.
“In robotics, people often claim that we don’t have enough training data,” says Lirui Wang, an electrical engineering and computer science graduate student at MIT who is working on the project. “But, another big problem is that the data come from so many different domains, modalities and robot hardware.
A robotic “policy” takes in sensor observations, like camera images or proprioceptive measurements that track the speed and position of a robotic arm, and then tells a robot how and where to move.
Policies are typically trained using imitation learning, meaning a human demonstrates actions or teleoperates a robot to generate data, which are fed into an AI model that learns the policy. Because this method uses a small amount of task-specific data, robots often fail when their environment or task changes.
To develop a better approach, Wang and his colleagues drew inspiration from large language models like GPT-4. These models are pretrained using an enormous amount of diverse language data and then fine-tuned by feeding them a small amount of task-specific data. Pretraining on so much data helps the models adapt to perform well on a variety of tasks.
“In the language domain, the data are all just sentences,” explains Wang. “In robotics, given all the heterogeneity in the data, if you want to pretrain in a similar manner, we need a different architecture.”
According to Wang, robotic data take many forms, from camera images to language instructions to depth maps. At the same time, each robot is mechanically unique, with a different number and orientation of arms, grippers and sensors. Plus, the environments where data are collected vary widely.
Wang and his colleagues at MIT’s Computer Science and Artificial Intelligence Laboratory developed a new architecture called Heterogeneous Pretrained Transformers that unifies data from these varied modalities and domains.
They put a machine-learning model known as a transformer into the middle of their architecture, which processes vision and proprioception inputs. A transformer is the same type of model that forms the backbone of large language models.
“Our dream is to have a universal robot brain that you could download and use for your robot without any training at all,” says Wang. “While we are just in the early stages, we are going to keep pushing hard and hope scaling leads to a breakthrough in robotic policies, like it did with large language models.”
BMW Assembly Plant Named ‘Factory of the Year’
REGENSBURG, Germany—BMW AG’s assembly plant here has been named the “Factory of the Year” (the European equivalent of the Assembly Plant of the Year award) by the Kearney consulting firm and the German trade magazine Produktion. The state-of-the-art facility, which produces X1 and X2 sport utility vehicles, was cited for its flexibility and digital production prowess.
“The innovative strength and dynamism with which the Regensburg plant is implementing the transformation to iFactory together with the entire workforce is impressive,” says Daniel Stengel, a director at Kearney and project manager for the competition. “In particular, the flexibility both in the factory setup and on the part of the employees is exemplary….”
The 38-year-old assembly plant also received praise for its use of artificial intelligence and other digital tools to improve productivity and quality.
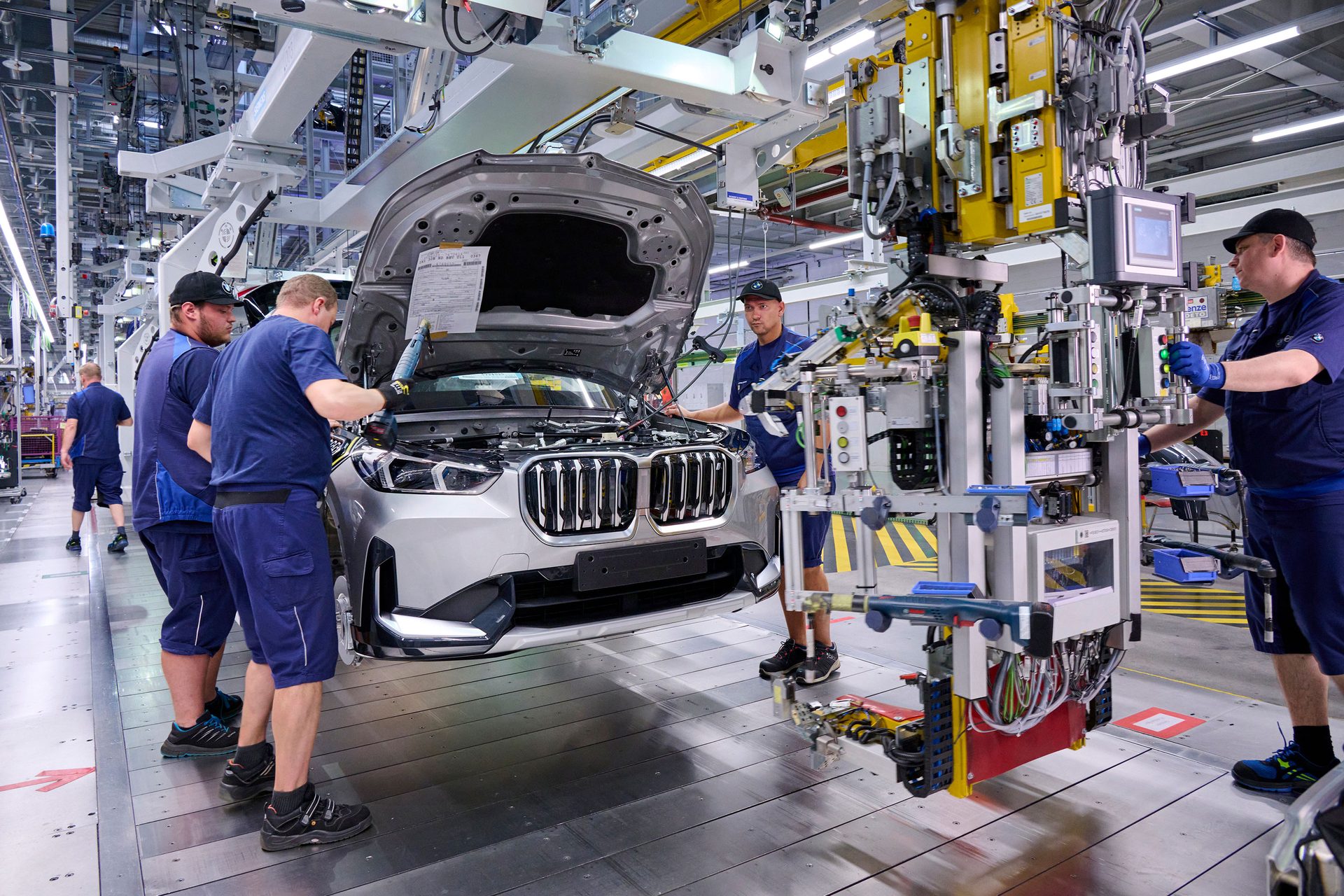
BMW’s state-of-the-art assembly plant in Regensburg, Germany, is extremely flexible. Photo courtesy BMW AG
According to Stengel, the logistics behind this process are extremely complex. The components and individual parts required for production always need to be in the right place at the right time, delivered just in time and just in sequence.
At BMW Regensburg, this logistical task is primarily handled by driverless, automated or autonomous transport systems that are intelligently connected through a cloud-based traffic control system known as BMW Automated Transport Services (ATS). In some cases, loading and unloading of transport vehicles is also fully automated.
Depending on the transport task, various types of driverless vehicles are used. For example, automated tugger trains transport small parts from the warehouse to the assembly line, while larger components weighing up to 1 ton are handled by smart transport robots.
These flat transport machines operate autonomously using dedicated AI modules. Relying on sensors and software, the devices recognize their surroundings, allowing them to navigate around obstacles independently when oncoming traffic permits.
The transport devices are managed through the central cloud-based BMW traffic control system. When it is time to replenish supplies, an employee on the assembly line rotates the change frame with the empty part containers and uses it to operate a retrieval rocker.
The control system then receives a signal indicating that new parts need to be delivered. It provides optimal route guidance, based on delivery priorities, and ensures transport devices are safely parked and their batteries sufficiently charged.
The Regensburg plant currently enables intelligent connection between nearly 50 automated tugger trains and more than 140 Smart Transport Robots through the control system. The fleet compromises both automated and autonomous devices from different manufacturers, handling approximately 10,000 part deliveries each workday.
In-Space Test Explores Cold Welding Benefits
CAPE CANAVERAL, FL—A new R&D project will study cold welding techniques for in-orbit spacecraft repairs. It’s an out-of-this-world idea akin to patching a tire while travelling through outer space.
The experiment recently arrived at the International Space Station (ISS) via SpaceX’s 31st Commercial Resupply Services mission for NASA.
The remotely controlled experiment, designed to address hull breaches caused by micrometeoroids or space debris, consists of four chambers with calibrated metallic samples. If successful, the project could establish cold welding as a crucial tool for in-space repair, significantly boosting the durability of spacecraft and the safety of crewed missions.
A new R&D project is studying cold welding techniques for in-orbit spacecraft repair. Photo courtesy International Space Station National Laboratory
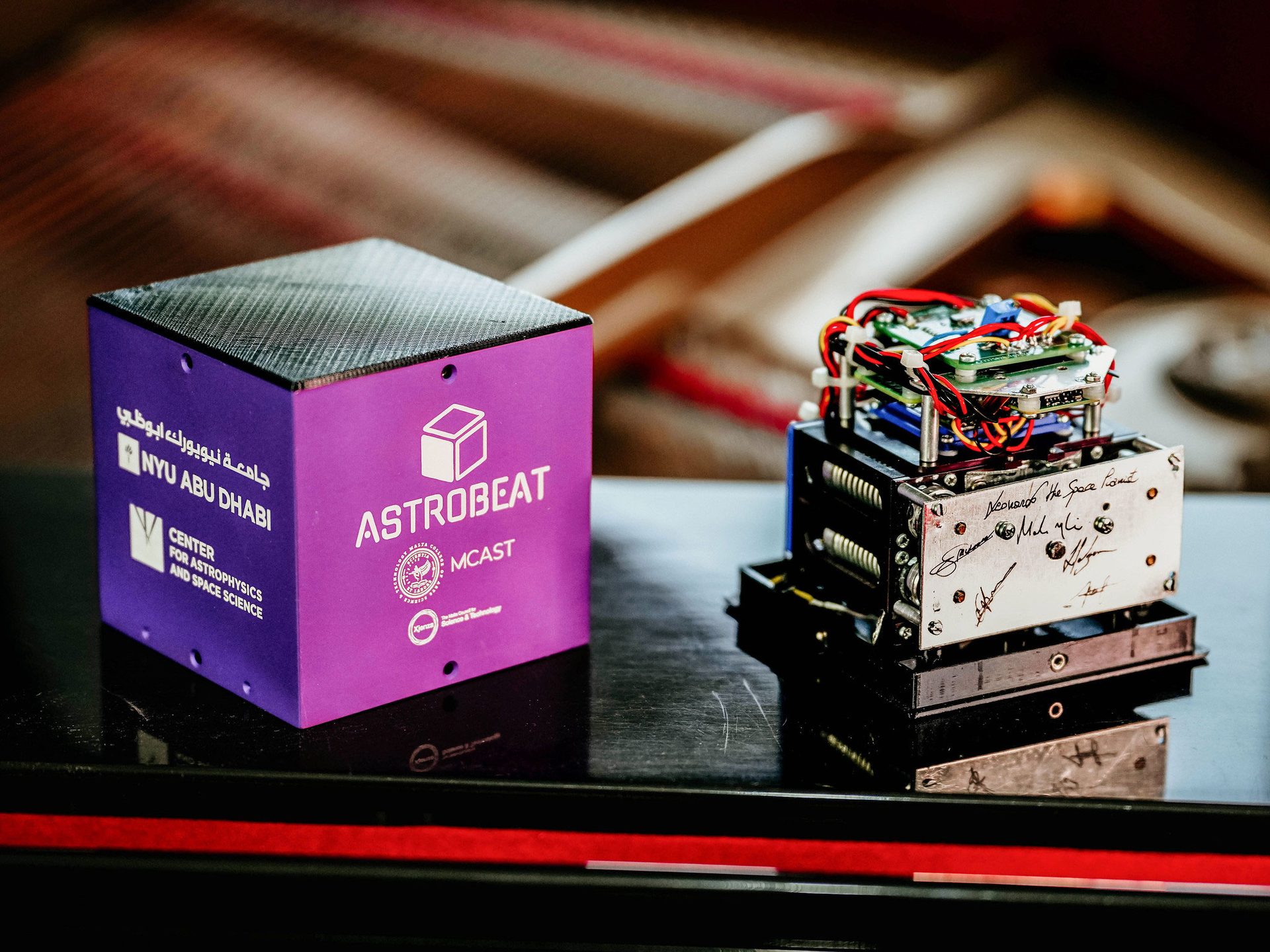
The Astrobeat initiative is spearheaded by Leonardo Barilaro, Ph.D., a senior lecturer in aerospace engineering at the Malta College of Arts, Sciences and Technology. The project will evaluate cold welding in space by testing metal patches on simulated spacecraft hulls, offering a safer and potentially more reliable method for in-orbit repairs than traditional techniques.
Cold welding uses a calibrated force to join metallic materials without heat, minimizing risks like structural damage to spacecraft from high temperatures. This technique benefits from the space station’s microgravity environment, where metals don’t re-oxidize quickly, allowing for cleaner and stronger bonds.
“Cold welding has long been considered an adverse phenomenon in space, but with Astrobeat, we’re transforming a problem into a viable repair mechanism,” says Barilaro. “It aims to create a paradigm shift in conducting structural repairs in space—swiftly, efficiently and with minimal external intervention.
“The introduction of Astrobeat to the ISS represents a crucial step from theoretical concepts to practical, potentially life-saving technology,” claims Barilaro. “[Our goal is] to provide astronauts with the capability to seal breaches from within spacecraft, leveraging the unique conditions of cold welding adhesion in space.”
Hyundai Reveals Smart Manufacturing Technologies
SEOUL—Hyundai Motor Group, the parent of automakers Hyundai and Kia, and supplier Hyundai Mobis, recently revealed the smart factory technologies that it’s deploying in assembly plants. The E-Forest Tech Day also presented the company’s vision for its future transition to a software-defined factory (SDF) system.
The event encouraged “technological exchange and mutual growth” among Hyundai Group companies and partners. Cutting-edge production technology on display included artificial intelligence, automated logistics and assembly tools, data twins and robotics.
“The focus of the event is to share and discuss pioneering technologies that we believe will shape the future of manufacturing plants,” says Jae Min Lee, vice president and head of E-Forest Center of Hyundai Motor and Kia. “This event is a crucial step in our ongoing efforts to incorporate these innovative technologies into our production facilities.
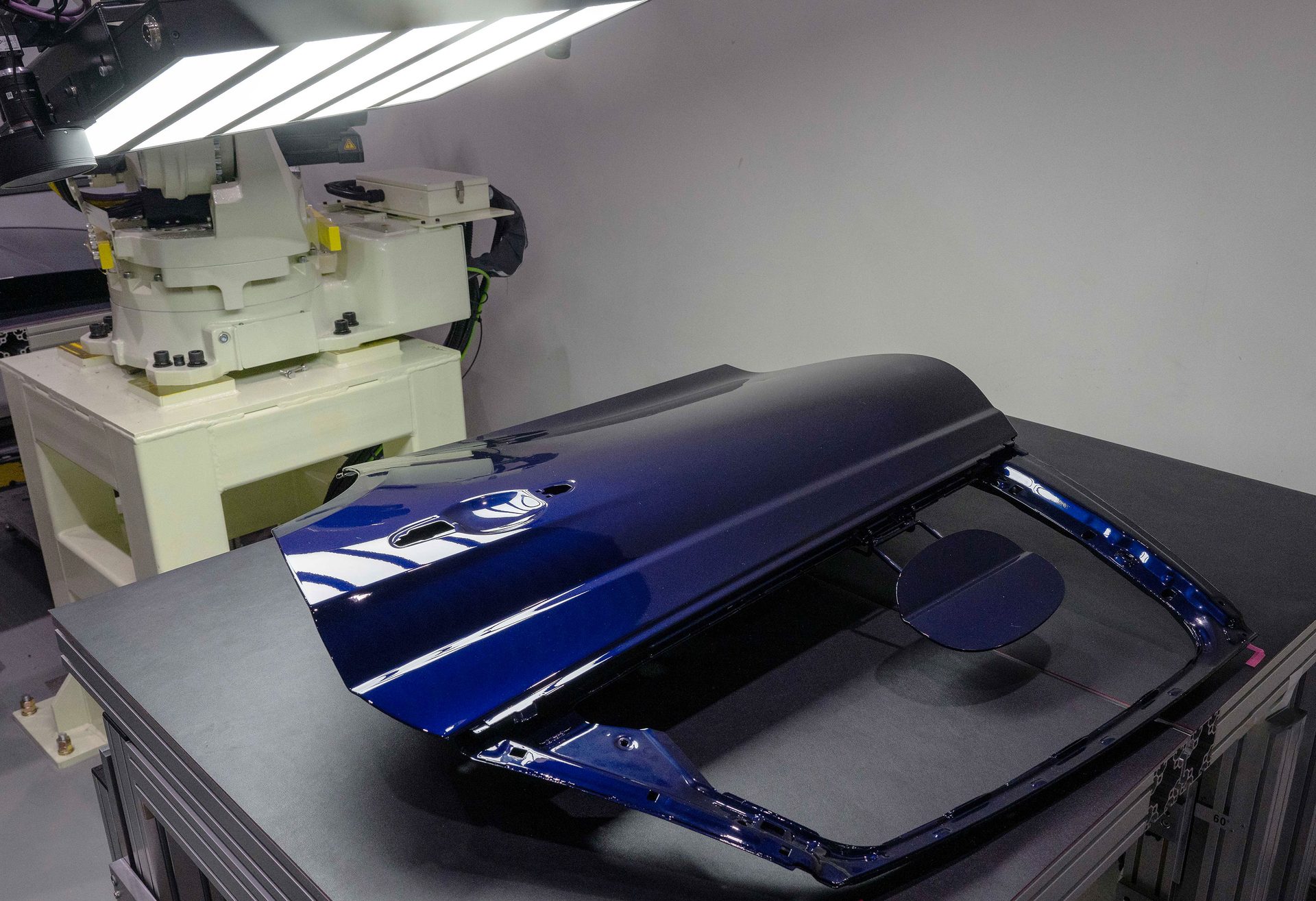
Hyundai Motor Group is deploying a variety of cutting-edge production technology to improve productivity and quality at its assembly plants. Photo courtesy Hyundai Motor Group
“The letter ‘E’ signifies a commitment to achieving progress in the entire mobility industry environment in a manner that is both efficient and economical,” explains Lee. “E-Forest, which SDF is based on, is set to become a production hub for software-defined vehicles. It aims to deliver products that cater to customer requirements faster than any other, through data connection and digital transformation.
“As the SDF is established in the future, enhanced manufacturing intelligence and increased flexibility will be achieved,” claims Lee. “This will enable data and software-based decision making and factory operations. The anticipated benefits include a shorter production preparation period, improved production speed, reduced investment costs for new vehicle introductions and enhanced quality.”
The initiative includes technology such as:
• Atypical Flexible Parts (HOSE) Automatic Assembly Technology. The program recognizes irregular parts, such as hoses and wires, through an AI vision algorithm. It automatically calculates the picking point and issues control commands. “This technology is essential for autonomous factory operations when humanoid robots are introduced into factories,” says Lee.
• Infinite Multi-Axis Holding Fixture. This tool is designed to improve flexibility in assembly plants. It allows various parts, such as doors, hoods and wheels, to be assembled using a single fixture. “When a part changes, the corresponding information is automatically input into the PC, automatically moving the fixture’s part fixing point and eventually fixing the part structure,” explains Lee.
• SPOT! Industry-Wide Solution. This technology can perform real-time safety inspections and equipment checks in factories. This is achieved by introducing intelligent inspection technology that uses AI, vision processing and big data processing. “Various sensors equivalent to eyes, nose and mouth are used in this technology,” says Lee.